I'm Dileep Kumar Gedela, former data engineer and a cloud architect with a deep love for transforming data into business insights. Over the past 5 years, I've had the privilege of working at Accenture, collaborating with world class technologists, and leveraging cutting-edge solutions to drive data solutions for various industries.
Data is an essential part of most modern businesses — used to propel growth, efficiency, and competitive advantage. The successful transformation of data into actionable insights heavily relies on the collaborative efforts of business stakeholders, data engineers, and business analysts. In my experience, however, this partnership often encounters challenges that impede the efficient execution of data initiatives.
In this blog article, we will explore common obstacles faced by data engineers, analysts, and business stakeholders.
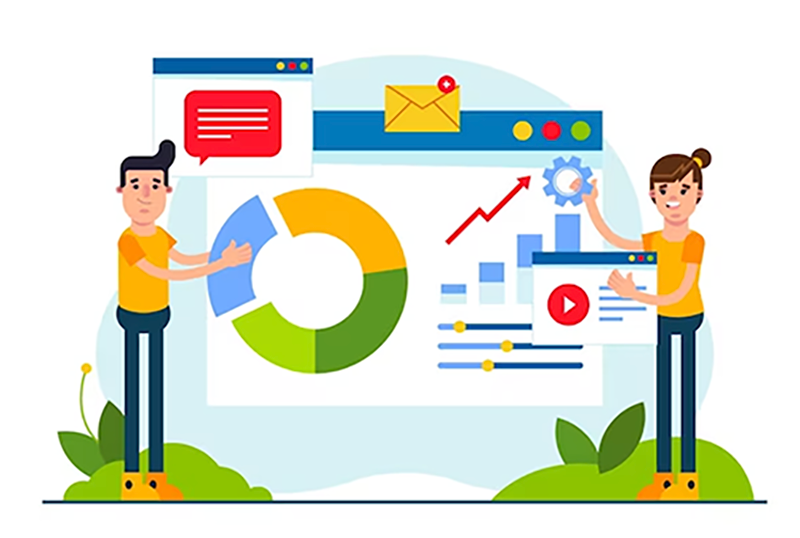
The Ideal Process vs. Reality:
As a data engineer, I believe that the analytics process starts with aligning all stakeholders on a problem and desired outcomes. This collaborative effort often sets the stage for core data engineering work of modeling and pipeline creation. Data modeling is the process of crafting a conceptual representation of an organization's data and its relationships, while data pipelines facilitate data ingestion and transformation, enabling data querying and analysis. Both activities require deep domain context. Ideally, everyone would be equipped with a clear understanding of the data’s structure and requirements, along with business context necessary to inform the underlying data work. However, the reality often deviates from this workflow. As data engineers, we find ourselves grappling with data complexities, trying to bridge the gap between expectations and the challenges we encounter.
The reason for this frequent disconnect lies in the complexity of real-world data scenarios. Data engineering is no simple task, and the mountains of data sources, formats, and quality variations add layers of intricacy to the process. Balancing the aspirations of business stakeholders with the technical realities of data integration and transformation is a continuous balancing act.
Why does this happen so frequently?
- Inadequate Cross-Functional Team Collaboration: When data engineers, analysts, and business stakeholders fail to communicate and collaborate effectively, it can result in misunderstandings and misaligned priorities. Data engineers might develop solutions without fully comprehending the business context, leading to insights that don't meet the specific needs of business stakeholders.
- Difficulty with Domain Context: Data engineering involves complex technical intricacies that may be unfamiliar to business stakeholders. This lack of technical understanding or context can give rise to unrealistic expectations and requirements, leading to data engineering efforts that fall short of expectations.
- Pressure from Time Constraints: The fast-paced nature of business environments often demands quick results. Deadlines can compel data engineers to rush through processes, potentially compromising data quality and accuracy in the pursuit of meeting deadlines.
What are the consequences that emerge from these challenges?
These obstacles can result in redundant efforts, inefficient pipelines and models, and bottlenecks that inevitably cause delays in project timelines. Such inefficiencies not only consume valuable time and resources, but also impede the overall efficiency and success of data initiatives. For organizations to be data-driven, bridging the gap between data teams and business stakeholders is crucial. There are many tools available, each catering to specific pain points in the analytics process. However, despite the array of solutions, analytics continue to be difficult and businesses struggle to capture value.
In my experience and view, the path to productive analytics process lies in fostering effective collaboration, clarity and understanding, and shared goals. Data teams and business stakeholders must work hand-in-hand to create efficient and effective data driven outcomes for their business. Coming full circle, this is one of the reasons that I decided to pursue my MBA at the University of Washington — to become an effective data-driven business leader.